Multivariate Student-t Posterior Predictive - Detailed Derivation
I'm a CS Ph.D. student wishing to improve my math skills by solving problems relevant to my research. I started this derivation of student-t posterior predictive some time ago and I keep getting stuck on it. I think I managed to go very far already, but I'm running out of time.
In the current exercise, I'm tackling a multi-variate posterior predictive with unknown mean and covariance, and multivariate outputs. I provide my notes with several pages of context and then towards the end you can find a spot I got stuck in. The line's unfinished, I tried "approach 2", some intermediate solutions on a side, but nothing works.
I know the solution is multivariate student's t and the problem is almost finished. But due to my background, I'm looking for someone who would locate potential mistakes and finish the solution in an extremely detailed way, preferably not taking massive shortcuts due to usage of "trace" and "determinant". I think the main issue I have is I constantly need to look up all the matrix operations to make sure what I do is kosher, and I'm probably missing some identities. For example, someone suggested a Woddbury formula.
So to be clear again - I'm offering a higher bounty primarily for the learning experience - not for the final solution. And I'm open to negotiate the price based on initial proposal.
Also my writing is horrible - don't hesitate to ask for clarifications!
Thanks!
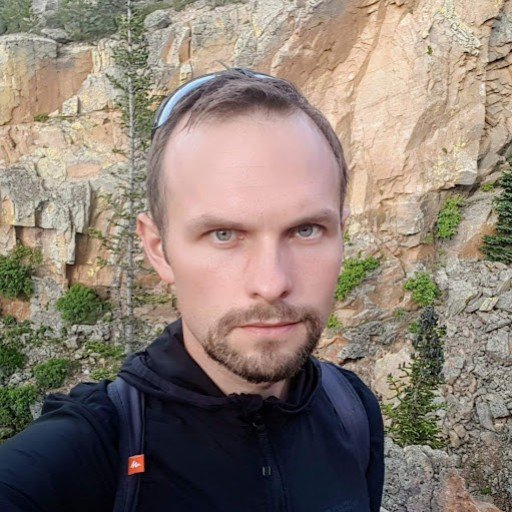
Answer
- The questioner was satisfied with and accepted the answer, or
- The answer was evaluated as being 100% correct by the judge.
1 Attachment
-
The fact that det(I+xx^T)=1+x^T x can be seen by noting that the eigenvectors of I+xx^T are x and the subspace orthogonal to x. The eigenvalues are then all 1 except one 1+x^Tx=1+|x|^2 . Let me know if you have any questions.
-
This was quite challenging and considerably more time consuming than it appeared at first. Please consider adding a tip if possible.
-
Sounds good. It looks solid at first look. Give me a day or so to review it and ask questions if I find anything unclear. And for sure, I'll leave you a tip if all makes sense to me. Thanks!
-
You're welcome. Sure, take your time.
-
-
Sorry for the delay. I just went through it all and it's fantastic! Exactly what I needed. There is something minor off with (1+xLambdax) raised to {-m/2} and then {-m/2} disappears in the next line when multiplied by det(S_n)^{-1/2}. I have a feeling det(S_n)^{-1/2} might have needed to be raised to {-m/2} somewhere earlier. If you have time you could take a look, but again - this is minor. It's great work overall and I'm happy to tip you for extra time you put. Cheers!
-
No problem. Thanks for the tip. The power of (1+x Lambda x) is meant to be -m/2 in the next line too. But the power of det(S_n) is correctly -1/2. The change in the power of det(S_n) comes from det(Psi) in the previous line. Also to get det(Sigma0)^-1/2 we need det(S_n)^-1/2 and (1+x Lambda x)^-m/2 as Sigma0 is an m by m matrix and (1+x Lambda x) is a scalar.
-
-
Ah. This is the main bit that I missed in the transition "(1+x Lambda x)^-m/2 as Sigma0 is an m by m matrix and (1+x Lambda x) is a scalar". Thanks!
-
You're welcome
-
- answered
- 406 views
- $150.00