Bayesian Statistics
Question for @Mathe
Hi, so next section which is unclear is Bayesian Statistics.
I don't know if I understand it right so please explain :)
Here we are choosing some random distribution calling it "prior" and set its parameters to some random values?
Then based on samples we get those parameters become more and more precise?
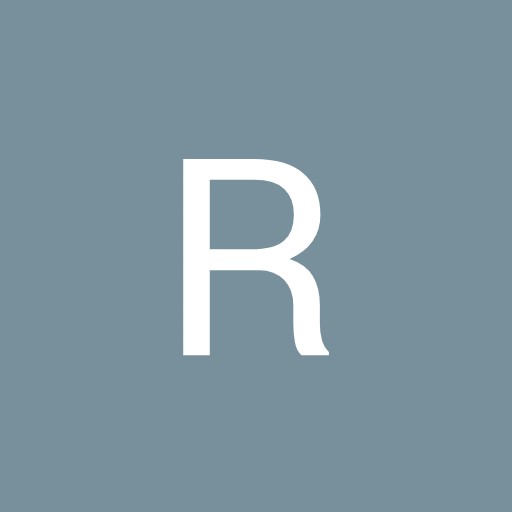
1 Answer
In bayesian statistics, there is a $p_{model}(x|\theta)$ which depends on an unknown parameter $\theta$ (or vector of parameters).
$\theta$ is treated like a random variable and has its own pdf called prior: $p_{prior}(\theta|\gamma)$. This pdf depends as well on parameters, in our case $\gamma$.
Here we are choosing some random distribution calling it "prior" and set its parameters to some random values?
Careful, we don't choose the prior distribution or its parameters "at random". We choose them making reasonable considerations and sometimes, even based on the sample of observed values. Once we have chosen the prior and the paramaters for the prior, they remain fixed!
Next, we compute a posterior density for $\theta$, $p_{posterior}(\theta|x)$, using Bayes rule. Notice that in this case, the observed values in the sample are constants. Almost like "fixed parameters". Bayes rule says: $$p_{posterior}(\theta|x) = \dfrac{p_{data}(x|\theta)p_{prior}(\theta)}{\int_\theta p_{data}(x|\theta)p_{prior}(\theta)}$$ Then based on samples we get those parameters become more and more precise?
Careful, it's not the parameters for the prior that get more precise. The information in the sample is combined with the information in the prior to give us a more precise understanding of the random variable $\theta$.
This information is contained in the posterior for $\theta$, and we might ask ourselves now things like what is the expected value of $\theta$ or what is its variance, etc.
Example:
Suppose we are interested in knowing the efficacy of a treatment to heal a person, that is, we are interested in the probability $p$ of a person being cured after following the treatment. We know that the treatment was administered to 100 patients, and 60 were cured.
Now, we want to follow a Bayesian approach, so we treat the unknown probability $p$ as a random quantity. Based on previous studies, we believe the efficacy of the treatment could be modeled by a beta distribution with parameters $\alpha = 3, \beta = 5$. We want to see how the recent sample of treated patients can update our beliefs about the treatment.
The prior then takes the form of $p_{prior}(p) \propto p^{3-1} (1-p)^{5-1}$ and the likelihood function is $p_{data}(x|p) \propto p^{60}(1-p)^{40}$.
This gives as a result a posterior of the form $p_{posterior}(x|p) \propto p^{60+3-1}(1-p)^{40+5-1}$. We recognize this posterior as a beta density with parameters $60+3$ and $40+5$. We can conclude that the mean value of $p$ is $\dfrac{60+3}{60+3+40+5}$
-
Thanks for explanation, could you please add an example for everything mentioned above, so I will understand it better please?
-
Yes, give me a few minutes.
-
I added an example.
-
-
In your example you describing the efficacy of the treatment could be modeled by a beta distribution with parameters α=3,β=5. The prior is a distribution which describes distribution of possible parameter values. So in this case parameters are α and β. So why pprior(p) describes distribution of parameters?
-
prior(p) does not describe the distribution of the parameters alpha and beta. Alpha and beta are fixed constants used in the prior, but they are not the object of interest.
-
so yeah, you treat probability p as object of interest, but If p is object of interest then p is a parameter. So it is part of some pmodel, but I can't imagine how probability can be a parameter of other probability distribution
-
pmodel is a Bernoulli density. In this model, p is a parameter.
-
-
@Mathe posted another question here https://matchmaticians.com/questions/uyoqfo so if you are interested you can answer
-
Yes, this is correct. Classic and bayesian statistics agree on the density pmodel. They disagree on the method of estimation. Classics used Maximum Likelihood, Bayesians build posteriores.
-
-
@Mathe Hi, I will tip you for answering my questions. In your example with treatment you have 100 samples with 60 success. AFAIK it can be modeled as Binomial distribution with 2 parameters (n - number of trials, and p - probability of success) Do I understand correctly that it can be used in Bayesian? From example above p is θ. We construct pprior to construct distribution for values p from Binomial can take? And pposterior is just more precise distribution which describes possible values of p?
-
Yes, you understood everything correctly. The situation I described can be modeled with a binomial distribution. This is used in a bayesian analysis as well. p is the parameter of interest, so the prior is chosen around the possible values p can take (from 0 to 1), and the posterior gives a more precise distribution of the possible values of p.
-
@Mathe But then it means that all Bayesian method does is estimating parameters of other distributions treating their parameters as random variables?
-
- 1 Answer
- 990 views
- Pro Bono
Related Questions
- Probability question dealing with expected value shown below
- Exponential Probability
- Probability
- Card riffle shuffling
- Find the maximum likelihood estimator
- Exponential random variables
- Probability maximum value of samples from different distributions
- Find Mean, Variance, and Distribution of Recursively Defined Sequence of Random Variables
Give me a few minutes.
I will tip you tomorrow morning. Good night!